Glossary terms:
- Types of data
- Qualitative data
- Quantitative data
- Levels of measurement
- Nominal
- Ordinal
- Interval
- Ratio
Classification of data
Welcome to Studypug's course in Statistics, on our first lesson we will learn about the methods for classification of data types since this will provide a useful introduction to the basics of this course, but before we enter into the concepts, do you know what is statistics?At this point you are well aware of what mathematics is, mathematics studies the world (and the universe for that matter) and looks for structure and patterns, and observes how they develop and change in space through time; mathematics' purpose is to make conjectures based on observations, just like any other science, but further than that, mathematics allows us to measure and translate our information gathered into a language that can be used universally to communicate our findings of the world.
How is this relevant to our lesson? Well, statistics is a branch of mathematics completely focused on gathering information, analyse and organize it and then present the findings of it. Every bit of information gathered is what we call data, and this could be anything, any group of things that are known, observed, measured, any group of facts which can be used to then perform calculations and prove hypotheses.
In simple words, statistics is the science in charge of collecting data, examine it, interpret it, organize it, and perform any mathematical operation necessary to then produce a certain result, a certain new characteristic of a population (any set of subjects, could be objects or people, or events) based on what was done. The main purpose of statistics is to be able to characterize a large population group based on the information gathered from a representative sample of it, in other words, statistical analysis allows us to use simple techniques to obtain information about a large group without having to scrutinize every single subject of the group, just a sample of them, making a study much more efficient.
We will focus more on what a statistical population is and how to obtain a representative sample of it in our next lesson about sampling methods. For now, let us focus on the topic of data classification, since this will tell us about the type of information that can be studied while doing an statistical analysis or research.
Qualitative data and quantitative data
There are two types of data in statistics: qualitative and quantitative.
On this section we will show how simple it is to define qualitative and quantitative data just by looking at the etymology of their names. It is probable that even if you have not heard about these concepts before, you may be able to infer on their significance given that the words "qualitative" and "quantitative" can be easily connected by our brains to the words they originate from: quality and quantity.
• Qualitative data
Given its characteristics, a qualitative variable is also called a categorical variable, and so, qualitative data is also called categorical data, since all you can do with such data type is to classify it or order it into categories of some sort.
From a sample of items, a qualitative study will gather information on variables such as the shape of the objects being studied, their color, the material they are made of, how are they called, etc.
The next list provides a few qualitative data examples:
- The vehicle models available in a car rental agency.
- The color of the houses in a neighborhood.
- The numbers on the shirts of hockey players.
- The breeds of puppies rescued in a shelter.
In short, qualitative data focus in descriptions and labels.
• Quantitative data
From a sample of items, quantitative variables can refer to the weight of the objects in the study, their temperature, their volume, or just about any type of measurement or numerical value from them.
There are two types of quantitative data: discrete and continuous.
- Discrete quantitative data refers to variables that can be counted and have a finite amount fixed.
- Continuous quantitative data is that which is measured and can have any value (even within a defined range).
For example, if you are to count the amount of people having dinner at a restaurant, this would be discrete data, first, because you are counting; second, you cannot have fractions of people, you can only have complete people. Discrete data comes in the form of whole numbers or integers.
On the other hand, if you measure the time it takes for each table in the restaurant to receive what they ordered (hopefully within the range of an hour) you will have values containing hours, minutes, second, and even fractions of a second if you want to increase precision! And so, these values would be a set of continuous quantitative data, first, because you measured them; second, because you can have any value (any value containing decimals, not just integers) within the reasonable range.
Having learnt this, we have a short list of quantitative examples of data:
- The amount of students in a classroom.
- The total amount of photographs saved on a memory card.
- The temperature on each day in spring.
- The weight of each person on a train.
Notice that from the four examples of quantitative variables listed above, the first two are examples of discrete variables, while the third and fourth are examples of continuous variables.
When learning about qualitative vs quantitative data, you will see in a lot of textbooks the quantitative definition tends to say this is the data type that uses numbers. On the special note in the next section you will see how the usage of numerical characters is not specific to quantitative data, but the usage of mathematical concepts and the usage of data values in numerical scales are.
What is the difference between qualitative and quantitative data
The main difference between qualitative and quantitative data is that you can count or measure a quantitative variable, while you can only describe or define the characteristics of a qualitative variable. When performing qualitative vs quantitative research the information that can be obtained is quite different, while qualitative data will provide the conditions of the study's subject (such as what type of object, color, shape, state, etc), quantitative data provides a amounts that have been counted or measured as the variables from the study's subject (such as how many incidences of an event per a unit of time, quantities such as weight, height, length, mass, and if the subject is moving, how fast and is it accelerating or not?).
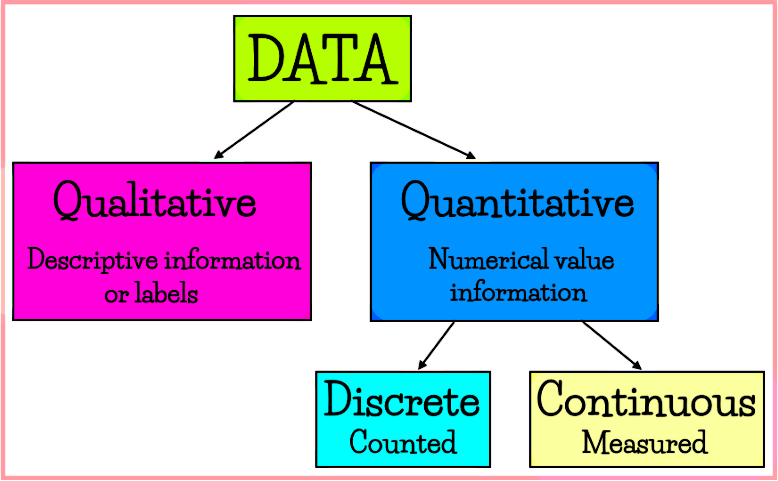
Numbers can also be used as a qualitative variable. Take for example your driver's license number or your student ID number, can you operate with them? No, but they are still numbers right? So how are they qualitative?
Well, these are numbers assigned to a particular person or thing (for example a car plates number), and so, they actually act like a name. For example, if you receive an student ID number of 012345, it means that the system within your school has you as "file 012345", and so, you could very well just go and say "I am 012345" and that would serve as another name to identify yourself for the school system. Thus, a student ID number is a label (quality) assigned to you, you cannot measure it, you cannot count it or operate on it and so, it cannot be quantitative.
The note above takes us to an important concept between quantitative vs qualitative data, which is the four levels of measurement of statistical data. In order from lowest to highest, the four levels of statistical data are nominal, ordinal, interval and ratio.
The word nominal comes from latin meaning "name", therefore, it is easy to understand that the nominal level of measurement for statistical data refers to names, labels or qualities. There is truly no numerical value and so the level of measurement is zero; therefore, statistical variables with only nominal level of measurement can be categorized in groups, but they cannot be arranged in any particular order.
Now, for the next data level of measurement, we know the term ordinal refers to the order of items in a list or series, and thus, this particular level of measurement refers to statistical data that can be ranked or ordered in a meaningful manner.
There is something very important to be said about data with an ordinal level of measurement, this type of data provides certain information that allow us to arrange the items in a particular order, but it does not provide any information about numerical values that the items on the list to be ordered might have. For example, if you were asked to rank a girl, her mom and her grandma from oldest to youngest, you would be able to do it without a problem because this data has an ordinal level of measurement (we can automatically infer that the grandmother is the oldest one, and the girl the youngest one because that is how nature works!) but you do not have any information about their ages, so you do not have any numerical information about them.
The interval level of measurement of a variable refers to an affine space, which is defined as an scaled space where the zero is the origin (the reference point of the settled system). In simple words, a variable has an interval level of measurement when it belongs to a scale range, be it physical scale spaces such as euclidean coordinate systems, or just significative scales such as the Celsius temperature scale.
For statistical data with interval level of measurement, the zero entry represents a position on the particular scale, but not an inherent value. For example, if a substance has a temperature of zero degrees celsius it does not mean that it has no heat, the zero point in the scale was picked because is the freezing point of water.
Notice that data with interval level of measurement is similar to data with an ordinal level of measurement in that they both can be ordered or ranked, the main difference is that data with interval level contains precise numerical value information in each of its tems.
The ratio level of measurement in statistical data is similar to the interval level, with the imperative difference that this kind of data has an inherent zero, meaning that the value of zero actually exists as a quantity, a variable of zero means "no quantity" or simply "none".
The term ratio is used since quantities are expressed as the ratio of the magnitude of a particular type of quantity against the quantity of the established unit in that scale; in simple terms, the ratio level of measurement is easily thought of "how many" or "how much" of a particular quantitative variable, and the value of zero truly means a value of none (this is what's called an inherent zero).
Remember that the Celsius scale of temperature was used to provide an example of the interval level of measurement, the Fahrenheit scale is also part of the interval level; the Kelvin scale on the other hand is not. The Kelvin scale has a value of zero which happens to be the absolute zero value of heat, meaning there is not kinetic energy (heat) in a body with such temperature (which by the way, is unobtainable); therefore, the Kelvin temperature scale has a level of measurement of ratio.
Examples of qualitative and quantitative data
On this section we will use the quantitative and qualitative data definitions we saw above and answer a few data classification examples.
Example 1
Determine which of the following data is quantitative or qualitative:
- The marks that students get in a test.
- The genders of newborn babies.
- The area codes in phone numbers.
- The heights of buildings.
Before continuing, identify the type of data by yourself and then look at the answers and explanations provided below:
- The marks that students get in a test. Answer: Quantitative.
- The genders of newborn babies. Answer: Qualitative.
- The area codes in phone numbers. Answer: Qualitative.
- The heights of buildings. Answer: Quantitative.
Test marks are numerical values that can be compared, and have an intrinsic value to them belonging to a scale, they are not labels. Therefore, this is quantitative data.
Gender is a descriptive variable, therefore, a study gathering this kind of information would be collecting qualitative data.
Although area codes are numbers, they are labels assigned to particular geographical areas within a city. They can be ordered, but cannot be counted or measured using the numerical symbols in them, thus, they are qualitative data.
The heights of buildings are numerical values that can be measured, even more, they can be any value within a reasonable range and so, this so happens to be continuous quantitative data.
Example 2
- The number of customers visiting a store over a weekend.
- The amount of water consumed by a country over the past 10 years.
- The outcomes of rolling a 6-sided die ten times.
- The heights of trees in a rainforest.
- Students' shoe sizes in a class.
Once more, make sure you identify which items in the above list are discrete and which are continuous before checking our answers and explanations below:
- The number of customers visiting a store over a weekend Answer: Discrete.
- The amount of water consumed by a country over the past 10 years. Answer: Continuous
- The outcomes of rolling a 6-sided die ten times. Answer: Discrete
- The heights of trees in a rainforest. Answer: Continuous
- Students' shoe sizes in a class. Answer: Discrete
You can count the number of customers in the store and the resulting quantities are whole numbers.
The amount of water can be measured and the resulting value will probably contain decimals, not just integers, since it requires higher levels of precision than just whole numbers.
There are just 6 possible outcomes of rolling a 6-sided die; since the possible outcomes are a finite number that can only be expressed in whole numbers, this is discrete data.
Measuring the heights of trees will result in values containing decimals, and these would be any value within the range of possible heights of a tree species.
Shoe sizes can belong only to a particular range of values, therefore, they are not continuous.
Example 3
Identify the level of measurement used in the following scenarios (nominal, ordinal, interval or ratio):
- A research on the causes of deaths in a country.
- A research that wants to find out the relationship between the amount of time students spend on preparing for the exam and the marks they get in it.
- A survey tries to find out how people rank the importance of: safety, price, speed, and comfort, when they are buying cars.
- A research on how humidity in the air changes over the year in a city.
Have you gotten your answers ready? Now check our answers and explanations below:
- A research on the causes of deaths in a country. Answer: Nominal
- A research that wants to find out the relationship between the amount of time students spend on preparing for the exam and the marks they get in it. Answer: Ratio
- A survey tries to find out how people rank the importance of: safety, price, speed, and comfort, when they are buying cars. Answer: Ordinal
- A research on how humidity in the air changes over the year in a city. Answer: Interval
This data comes from qualitative research which will provide the descriptions of what is causing deaths in the population of a country, therefore each variable will be a labeled category only, thus nominal.
This data comes from quantitative research that can have an inherent zero among the values of the statistical data gathered. Simply said, a student could have spent no time studying, therefore, this data has a level of measurement of ratio.
This data can be ranked without having numerical values for each variable, therefore its level of measurement is ordinal.
The key point to determine the level of measurement of this data is to observe that the data is collected as values (thus is quantitative data) belonging to a particular range of values, an affine space, where the zero is not included, and so, this data has an interval level of measurement.