Introduction to normal distribution
Intros
Lessons
Examples
Lessons
- The Normal Distribution and the 68-95-99.7 Rule
The weight of chocolate bars produced by a factory is normally distributed with a mean of 225 grams and a standard deviation of 5 grams. Determine the percentage of the chocolate bars that could be expected to weigh
a) between 220 and 230 grams.
b) between 215 and 235 grams.
c) between 210 and 240 grams.
d) between 225 and 230 grams.
e) between 230 and 235 grams.
f) between 210 and 215 grams.
g) between 220 and 240 grams.
h) above 225 grams.
i) above 240 grams.
j) below 220 grams.
- Using "invNorm" to Solve Normal Distribution Problems
The weight of chocolate bars produced by a factory is normally distributed with a mean of 225 grams and a standard deviation of 5 grams.- The factory has an acceptable minimum weight and all chocolate bars below the minimum weight standard are rejected. If 7% of the chocolate bars are rejected, determine the acceptable minimum weight.
- If the heaviest 10% of the chocolate bars are classified as "Supersize Chocolate Bars", what is the weight of the lightest Supersize Chocolate Bar?
Free to Join!
Easily See Your Progress
We track the progress you've made on a topic so you know what you've done. From the course view you can easily see what topics have what and the progress you've made on them. Fill the rings to completely master that section or mouse over the icon to see more details.Make Use of Our Learning Aids
Earn Achievements as You Learn
Make the most of your time as you use StudyPug to help you achieve your goals. Earn fun little badges the more you watch, practice, and use our service.Create and Customize Your Avatar
Play with our fun little avatar builder to create and customize your own avatar on StudyPug. Choose your face, eye colour, hair colour and style, and background. Unlock more options the more you use StudyPug.
Topic Notes
Introduction to Normal Distribution
Welcome to our exploration of the normal distribution, a fundamental concept in statistics. This bell-shaped curve is a cornerstone of data analysis, describing many natural phenomena. Our introduction video offers a clear, visual explanation of this crucial statistical concept. You'll discover why the normal distribution is so prevalent and how it applies to real-world scenarios. The video breaks down complex ideas into digestible segments, making it perfect for students new to statistics. You'll learn about key features like the mean and standard deviation, and how they shape the curve. Understanding the normal distribution is essential for interpreting data, making predictions, and conducting statistical tests. Whether you're a beginner or looking to refresh your knowledge, this video provides a solid foundation. By the end, you'll appreciate why the normal distribution is often called the "bell curve" and its significance in various fields, from psychology to economics.
Understanding the Bell Curve
The bell curve, also known as the normal distribution, is a fundamental concept in statistics that helps us understand how data is distributed around a central value. To illustrate this concept, let's consider the example of human height, as mentioned in the video. Imagine we were to measure the height of every adult in a large population and plot this data on a graph. The resulting shape would closely resemble a bell, hence the term "bell curve."
At the center of the bell curve is the mean, which represents the average height of the population. In our height example, this might be around 5'9" (175 cm) for men or 5'4" (162 cm) for women. The key characteristic of the bell curve is that data points tend to cluster around this mean value. This means that a large proportion of the population will have heights close to the average.
As we move away from the mean in either direction, we observe a gradual decrease in the frequency of data points. This is represented by the sloping sides of the bell shape. For instance, there are fewer people who are extremely tall (e.g., 7 feet) or extremely short (e.g., 4 feet) compared to those of average height. The further we move from the center, the less common these height measurements become.
The symmetrical nature of the bell curve is another important feature. It indicates that deviations above and below the mean occur with roughly equal frequency. In our height example, this means there are approximately as many people who are 2 inches taller than average as there are people who are 2 inches shorter.
Understanding the normal distribution is crucial in statistics for several reasons. Firstly, it provides a model for many natural phenomena and human characteristics, not just height but also things like IQ scores, blood pressure readings, and measurement errors. This makes it a powerful tool for analyzing and predicting data in various fields, from social sciences to engineering.
Secondly, the bell curve allows statisticians to make inferences about a population based on a sample. By understanding how data is distributed, we can estimate the likelihood of certain outcomes or characteristics occurring within a population. For example, we can calculate the percentage of people likely to fall within a specific height range.
Moreover, the normal distribution forms the basis for many statistical tests and methods. Concepts like standard deviation, which measures the spread of data around the mean, are derived from the properties of the bell curve. These tools are essential for hypothesis testing, confidence intervals, and other statistical analyses used in research and decision-making processes.
In conclusion, the bell curve is a powerful visual and mathematical representation of how data is distributed in many real-world scenarios. Its shape, with data clustering around the mean and frequency decreasing as we move away from the center, provides valuable insights into the nature of variability within populations. By understanding this concept, we gain a fundamental tool for interpreting data, making predictions, and drawing meaningful conclusions across a wide range of disciplines.
Properties of Normal Distribution
The normal distribution, also known as the Gaussian distribution, is a fundamental concept in statistics and probability theory. It is characterized by several key properties that make it a powerful tool for analyzing and interpreting data across various fields. Understanding these properties is crucial for anyone working with statistical analysis or data science.
One of the most notable properties of the normal distribution is its symmetry. The bell-shaped curve is perfectly symmetrical around its central point, which means that the left and right sides of the curve are mirror images of each other. This symmetry is a defining feature of the normal distribution and has important implications for data analysis.
The mean, median, and mode are three essential measures of central tendency in statistics, and in a normal distribution, they all coincide at the same point. This point is located at the center of the distribution and represents the peak of the bell curve. For example, if we consider the distribution of human heights in a population, the mean height would be the average of all heights, the median would be the middle value when all heights are arranged in order, and the mode would be the most frequently occurring height. In a perfectly normal distribution of heights, all three of these values would be the same.
The standard deviation is another crucial property of the normal distribution. It measures the spread or dispersion of data points around the mean. In the context of the normal distribution, the standard deviation directly affects the shape of the curve. A smaller standard deviation results in a taller and narrower bell curve, indicating that the data points are clustered more closely around the mean. Conversely, a larger standard deviation leads to a flatter and wider curve, suggesting that the data points are more spread out.
To illustrate this concept, let's consider the distribution of IQ scores, which are designed to follow a normal distribution. The mean IQ is set at 100, with a standard deviation of 15 points. This means that approximately 68% of the population falls within one standard deviation of the mean (between 85 and 115), 95% within two standard deviations (between 70 and 130), and 99.7% within three standard deviations (between 55 and 145).
Another example is the distribution of adult weights in a population. If the mean weight is 70 kg with a standard deviation of 10 kg, we would expect about 68% of adults to weigh between 60 kg and 80 kg (one standard deviation from the mean), 95% to weigh between 50 kg and 90 kg (two standard deviations), and 99.7% to weigh between 40 kg and 100 kg (three standard deviations).
The normal distribution also exhibits the property of probability density. The total area under the curve is always equal to 1, representing 100% of the data. This property allows us to calculate the probability of a value falling within a specific range by finding the area under the curve for that range.
In addition to symmetry and the relationship between mean, median, and mode, the normal distribution has other important properties. For instance, about 68% of the data falls within one standard deviation of the mean, 95% within two standard deviations, and 99.7% within three standard deviations. This is known as the empirical rule or the 68-95-99.7 rule and is particularly useful for quick estimations and data interpretation.
The normal distribution's properties make it an invaluable tool in many fields. In psychology, it's used to analyze IQ scores and personality traits. In biology, it helps in understanding variations in physical characteristics like height and weight. In finance, it's applied to model stock price movements and assess investment risks. The versatility and mathematical tractability of the normal distribution have made it a cornerstone of statistical analysis and modeling across diverse disciplines.
The 68-95-99.7 Rule
The 68-95-99.7 rule, also known as the empirical rule or three-sigma rule, is a fundamental concept in statistics that describes the distribution of data in a normal distribution. This rule provides a quick and easy way to understand how data is spread around the mean in terms of standard deviations. Let's explore this rule in detail using the height example from the video.
Imagine we have a large dataset of adult male heights, which follows a normal distribution. The mean height is 70 inches (about 178 cm), and the standard deviation is 3 inches (about 7.6 cm). The 68-95-99.7 rule tells us about the percentage of data that falls within one, two, and three standard deviations from the mean.
First, let's look at one standard deviation:
- 68% of the data falls within one standard deviation of the mean
- In our height example, this means 68% of adult males have heights between 67 inches (70 - 3) and 73 inches (70 + 3)
- This range covers the majority of the population
Moving on to two standard deviations:
- 95% of the data falls within two standard deviations of the mean
- For our height example, 95% of adult males have heights between 64 inches (70 - 6) and 76 inches (70 + 6)
- This range encompasses a vast majority of the population
Finally, let's consider three standard deviations:
- 99.7% of the data falls within three standard deviations of the mean
- In our height example, 99.7% of adult males have heights between 61 inches (70 - 9) and 79 inches (70 + 9)
- This range covers almost the entire population, with only extremely rare cases falling outside
To help visualize this concept, imagine a bell curve representing the height distribution. The peak of the curve is at 70 inches (the mean), and as you move away from the center, the curve slopes downward. The areas under the curve correspond to the percentages mentioned in the 68-95-99.7 rule.
Understanding this rule is crucial for data analysis and interpretation. It allows us to quickly assess the spread of data and identify outliers. For instance, if we encounter a man who is 80 inches tall, we can recognize that this is an unusual height, falling outside three standard deviations from the mean.
The 68-95-99.7 rule applies to any normally distributed data, not just height. It could be used for analyzing test scores, product measurements, or any other dataset that follows a normal distribution. This makes it a versatile tool in various fields, from education to manufacturing quality control.
In summary, the 68-95-99.7 rule provides a simple yet powerful framework for understanding data distribution. By knowing that approximately 68% of data falls within one standard deviation, 95% within two, and 99.7% within three, we can quickly grasp the spread of data in a normal distribution. This knowledge is invaluable for interpreting data, identifying unusual values, and making informed decisions based on statistical analysis.
Real-World Applications of Normal Distribution
The normal distribution, also known as the Gaussian distribution, is a fundamental concept in statistics with numerous real-world applications across various fields. Beyond the examples provided in the video, this statistical model plays a crucial role in quality control, social sciences, and natural phenomena.
In quality control, manufacturers rely heavily on normal distribution to maintain product consistency and reliability. For instance, in the production of electronic components, the normal distribution helps predict the likelihood of defects within a batch. By understanding the distribution of measurements, companies can set acceptable tolerance levels and implement efficient quality assurance processes. This application ensures that products meet specified standards and reduces waste in manufacturing.
Social sciences extensively utilize normal distribution to analyze and interpret data related to human behavior and societal trends. In psychology, researchers often assume that many traits, such as IQ scores or personality characteristics, follow a normal distribution within a population. This assumption allows for meaningful comparisons and the development of standardized tests. Similarly, in economics, normal distribution aids in modeling financial market behaviors, risk assessment, and economic forecasting.
Natural phenomena frequently exhibit patterns that align with normal distribution. In biology, the distribution of physical traits within a species, such as height or weight, often follows a bell curve. Meteorologists use normal distribution to analyze and predict weather patterns, including temperature variations and precipitation levels. Geologists apply this concept to study the distribution of mineral deposits and assess the probability of natural disasters like earthquakes.
Understanding normal distribution in these contexts offers several benefits. It allows for accurate predictions and informed decision-making based on probability. Researchers and professionals can identify outliers or anomalies that may require further investigation. Moreover, it facilitates the development of standardized measures and benchmarks across different fields.
In conclusion, the applications of normal distribution extend far beyond theoretical statistics. From ensuring product quality to understanding human behavior and natural processes, this statistical model provides a powerful tool for analysis and prediction in diverse real-world scenarios. By recognizing the prevalence of normal distribution in various fields, professionals can make more informed decisions and gain deeper insights into complex phenomena.
Calculating Probabilities Using Normal Distribution
Understanding how to calculate probabilities using normal distribution is a fundamental skill in statistics and data analysis. The normal distribution, also known as the Gaussian distribution, is a symmetrical, bell-shaped curve that represents the distribution of many natural phenomena. This powerful tool allows us to determine the likelihood of certain events occurring within a given dataset.
At the heart of working with normal distributions are z-scores, which are essential for standardizing values and making comparisons across different datasets. A z-score represents the number of standard deviations a data point is from the mean of the distribution. By converting raw scores to z-scores, we can easily interpret the relative position of any value within the distribution.
To calculate a z-score, we use the formula: z = (x - μ) / σ, where x is the raw score, μ is the mean of the distribution, and σ is the standard deviation. This transformation allows us to work with a standard normal distribution, which has a mean of 0 and a standard deviation of 1.
Once we have converted our values to z-scores, we can use standard normal distribution tables or statistical software to find probabilities. These probabilities represent the area under the normal curve, which corresponds to the likelihood of certain outcomes.
Let's explore some simple examples of probability calculations:
1. Calculating the probability of a value being above a certain point: Suppose we want to find the probability of a randomly selected value being greater than 1 standard deviation above the mean. We would look up the z-score of 1 in a standard normal table, which gives us approximately 0.8413. This means there's about a 15.87% chance (1 - 0.8413) of a value being more than 1 standard deviation above the mean.
2. Finding the probability of a value falling below a specific point: If we need to determine the likelihood of a value being less than 2 standard deviations below the mean, we'd use a z-score of -2. The standard normal table shows this probability is about 0.0228, or 2.28%.
3. Calculating the probability between two points: To find the probability of a value falling between the mean and 1 standard deviation above it, we'd subtract the probability at z=0 (0.5) from the probability at z=1 (0.8413), giving us 0.3413 or 34.13%.
These calculations become particularly useful in real-world scenarios. For instance, in quality control, manufacturers might use normal distribution to determine the probability of a product meeting certain specifications. In finance, analysts may use it to assess the likelihood of stock price movements. Educational institutions often employ normal distribution to standardize test scores and evaluate student performance relative to their peers.
It's important to note that while many phenomena follow a normal distribution, not all do. Before applying these probability calculations, it's crucial to verify that your data is indeed normally distributed or can be reasonably approximated by a normal distribution.
As you become more comfortable with z-scores and probability calculations, you'll find that the normal distribution is an invaluable tool for making informed decisions based on data. Whether you're analyzing market trends, conducting scientific research, or evaluating process outcomes, understanding how to calculate probabilities using normal distribution will enhance your ability to interpret and predict outcomes in various fields.
Conclusion
In summary, this article has covered the essential aspects of normal distribution, a fundamental concept in statistics. We explored its bell-shaped curve, symmetry, and the significance of standard deviation. The introduction video provided a visual representation, making these abstract concepts more tangible. Key points include the 68-95-99.7 rule, z-scores, and the central limit theorem. Understanding normal distribution is crucial for data analysis, hypothesis testing, and making inferences about populations. The video's illustrations reinforced how this distribution applies to real-world scenarios. As you've grasped these basics, we encourage you to delve deeper into more advanced topics. Explore areas like skewness, kurtosis, and how normal distribution relates to other probability distributions. Additionally, investigate its applications in fields such as finance, psychology, and quality control. By expanding your knowledge, you'll enhance your statistical analysis skills and gain valuable insights into data interpretation.
Understanding the significance of these concepts is crucial for further studies. The central limit theorem plays a vital role in many statistical methods. Moreover, mastering hypothesis testing will allow you to make more accurate predictions and decisions based on data.
Using "invNorm" to Solve Normal Distribution Problems
Using "invNorm" to Solve Normal Distribution Problems
The weight of chocolate bars produced by a factory is normally distributed with a mean of 225 grams and a standard deviation of 5 grams.
The factory has an acceptable minimum weight and all chocolate bars below the minimum weight standard are rejected. If 7% of the chocolate bars are rejected, determine the acceptable minimum weight.
Step 1: Understand the Problem
The first step in solving this problem is to understand the given information and what is being asked. The weight of chocolate bars is normally distributed with a mean (μ) of 225 grams and a standard deviation (σ) of 5 grams. The factory rejects chocolate bars that are below a certain minimum weight. We need to determine this minimum weight such that 7% of the chocolate bars are rejected.
Step 2: Visualize the Normal Distribution
Since the problem involves a normal distribution, it is helpful to visualize the normal curve. The mean of 225 grams is at the center of the curve, and the standard deviation of 5 grams determines the spread of the distribution. The x-axis represents the weight of the chocolate bars, with weights increasing as you move to the right and decreasing as you move to the left.
Step 3: Identify the Area Under the Curve
In a normal distribution, the area under the curve represents probabilities. We are given that 7% of the chocolate bars are rejected, which means that the area to the left of the minimum acceptable weight is 0.07 (7%). This area represents the probability that a chocolate bar will be below the minimum acceptable weight.
Step 4: Use the invNorm Function
To find the minimum acceptable weight, we use the invNorm function, which calculates the value corresponding to a given cumulative probability in a normal distribution. The invNorm function requires three inputs: the cumulative probability (area to the left), the mean, and the standard deviation.
Step 5: Calculate the Minimum Weight
Using a calculator or statistical software, input the following values into the invNorm function:
- Cumulative probability (area to the left): 0.07
- Mean (μ): 225 grams
- Standard deviation (σ): 5 grams
Step 6: Interpret the Result
The result from the invNorm function gives us the minimum acceptable weight. This weight is the threshold below which chocolate bars are rejected. Ensure that the result makes sense in the context of the problem. Since the mean weight is 225 grams, the minimum acceptable weight should be less than 225 grams.
Step 7: Verify the Solution
To verify the solution, you can use the normal cumulative distribution function (normal CDF) to check that the area to the left of the calculated minimum weight is indeed 0.07. This step ensures that the invNorm function was used correctly and that the result is accurate.
Conclusion
By following these steps, you can determine the minimum acceptable weight for the chocolate bars using the invNorm function. This method is applicable to any problem involving normally distributed data where you need to find a value corresponding to a given cumulative probability.
FAQs
-
What is the normal distribution?
The normal distribution, also known as the Gaussian distribution, is a symmetrical, bell-shaped curve that represents the distribution of many natural phenomena. It's characterized by its mean (average) and standard deviation (measure of spread). The normal distribution is widely used in statistics due to its prevalence in real-world data and its mathematical properties.
-
What is the 68-95-99.7 rule in normal distribution?
The 68-95-99.7 rule, also known as the empirical rule, states that in a normal distribution: approximately 68% of data falls within one standard deviation of the mean, 95% within two standard deviations, and 99.7% within three standard deviations. This rule helps in quickly understanding the spread of data in a normal distribution.
-
How is the normal distribution used in real-world applications?
The normal distribution is used in various fields. In quality control, it helps predict defects in manufacturing. In social sciences, it's used to analyze human traits and behaviors. In finance, it aids in risk assessment and market analysis. Natural phenomena like height distributions and weather patterns often follow normal distributions, making it useful in biology and meteorology.
-
What is a z-score and how is it used in normal distribution?
A z-score represents the number of standard deviations a data point is from the mean in a normal distribution. It's calculated using the formula: z = (x - μ) / σ, where x is the raw score, μ is the mean, and σ is the standard deviation. Z-scores are used to standardize values, making it easier to compare data from different normal distributions and calculate probabilities.
-
How do you calculate probabilities using the normal distribution?
To calculate probabilities using the normal distribution, first convert the raw scores to z-scores. Then, use standard normal distribution tables or statistical software to find the area under the curve corresponding to these z-scores. This area represents the probability. For example, to find the probability of a value being less than a certain point, you would look up the z-score for that point in a standard normal table.
Prerequisite Topics for Introduction to Normal Distribution
Understanding the foundations of statistics is crucial when delving into the world of normal distribution. Before embarking on this journey, it's essential to grasp several key concepts that form the building blocks of statistical analysis.
One of the most fundamental prerequisites is the understanding of mean and standard deviation. These measures of central tendency and dispersion are vital in comprehending the shape and characteristics of normal distribution. The mean provides the center point of the distribution, while the standard deviation indicates how spread out the data is from this center. Mastering these concepts in the context of binomial distribution lays a solid groundwork for exploring normal distribution.
Another critical prerequisite is the central limit theorem. This theorem is a cornerstone in statistics, bridging the gap between various distributions and the normal distribution. It explains why many real-world phenomena tend to follow a normal distribution, making it an indispensable concept for students approaching the topic of normal distribution. Understanding this theorem helps in appreciating the widespread applicability and importance of normal distribution in statistical analysis.
While it might seem less directly related, familiarity with hypothesis testing methods such as Chi-Squared testing is also beneficial. These methods often rely on assumptions of normality, and understanding how they work can provide valuable context for the practical applications of normal distribution in statistical inference.
The normal distribution, often referred to as the Gaussian distribution, is a symmetrical, bell-shaped curve that is ubiquitous in statistics. Its properties and applications are deeply rooted in the concepts covered in these prerequisite topics. For instance, the mean and standard deviation directly influence the shape and position of the normal curve. The central limit theorem explains why so many natural phenomena approximate a normal distribution, making it a powerful tool in various fields of study and research.
Moreover, the normal distribution plays a crucial role in hypothesis testing, including more advanced methods like Chi-Squared tests. Understanding how these tests work and their underlying assumptions about normality can greatly enhance one's grasp of the normal distribution's significance in real-world statistical analysis.
By thoroughly exploring these prerequisite topics, students can build a strong foundation for understanding the intricacies of normal distribution. This knowledge not only aids in grasping the theoretical aspects but also in applying these concepts to practical scenarios in fields ranging from social sciences to natural sciences, economics, and beyond. The interconnectedness of these topics highlights the importance of a comprehensive approach to learning statistics, where each concept builds upon and reinforces the others.
⋅ About 68% of the population are within 1 standard deviation of the mean.
⋅ About 95% of the population are within 2 standard deviations of the mean.
⋅ About 99.7% of the population are within 3 standard deviations of the mean.
.
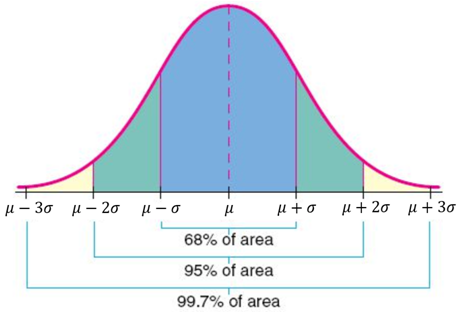
Calculator Commands
⋅ To calculate the normal distribution probability between two data values:
normalcdf (lower bound, upper bound, mean, standard deviation)
- To calculate the area to the left of a data value, replace the lower bound by −1×1099
- To calculate the area to the right of a data value, replace the upper bound by 1×1099
⋅ To calculate a data value, given the area to the left of the data value:
invNorm (area, mean, standard deviation)
remaining today
remaining today